
Since TFs recognize their target sites not only using hydrogen bonds but also using their structural fit, TF-binding motifs show preferences depending on k-mer words ( 13), particularly in the flanking regions outside the hydrogen bonding core region ( 14). The information on the geometric orientation of the bases propagates within the DNA for several positions before fading out, creating a dependence of the DNA physical properties on nucleotide pairs, triplets and longer k-mers. One reason is that the stacked, neighboring bases largely determine the physical properties of DNA, such as their equilibrium bending angle, minor groove width, propeller twist or helical twist. However, modeling the nucleotide inter-dependency often yields better motif predictions than PWMs ( 10–12). The PWM model has been enormously successful because for the vast majority of transcription factors it achieves quite high accuracy for predicting the binding affinity of high-affinity binding sites with only 3 W parameters for a binding site of W nucleotides. By Boltzmann’s law, this is equivalent to assuming statistical independence between nucleotides at different positions of the binding site.
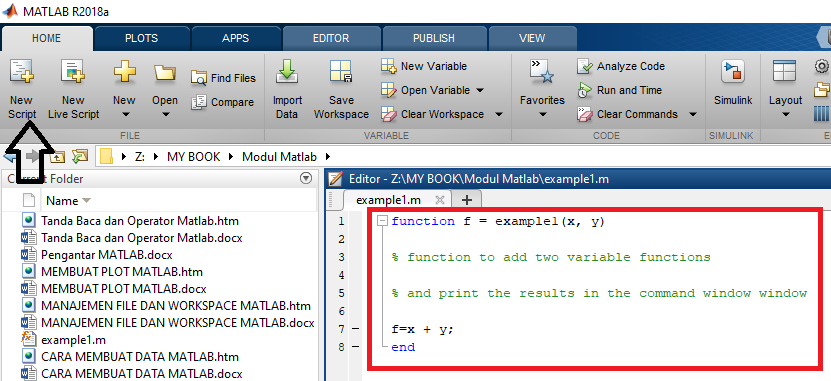
This model assumes that the binding energy can be decomposed into a sum of contributions from each of the nucleotides in the binding site. The dominant model for describing the binding affinity of transcription factors to DNA target sequences has been the position weight matrix (PWM). These measurements result in sets of hundreds to millions of bound sequences from which the binding motif model is deduced based on the statistical enrichment of binding sites compared to a background set of unbound sequences or a background model for random sequences. Common in vivo techniques are ChIP-seq ( 5) and bacterial-one-hybrid ( 6), while most modern in vitro approaches are SELEX-based ( 7–9). Motif models can be inferred from numerous types of experiments ( 4). The task of de novo motif discovery is to infer from experimental data a statistical or thermodynamic model that can then predict the binding affinity of a TF of interest for any sequence up to a constant (see Supplementary Methods subsection S1.2). Learning quantitative models from experimental data that allow us to accurately predict the binding affinities of TFs to any given sequence is important for quantitatively predicting transcription rates from regulatory sequences. These binding motifs typically contain 6 to 12 only partially conserved bases ( 1–3). Gene expression is regulated through the binding of transcription factors (TFs) to specific recognition motifs within promoter and enhancer DNA sequences. These results demonstrate that dependencies beyond first order clearly improve binding models for most TFs. BaMMmotif2 models showed no signs of overtraining in cross-cell line and cross-platform tests, with similar improvements on the next-best tool. BaMMmotif2 models of fifth-order achieved a median false-discovery-rate-averaged recall 13.6% and 12.2% higher than the next best tool on 427 ChIP-seq datasets and 164 HT-SELEX datasets, respectively, while being 8 to 1000 times faster. We tested it with state-of-the-art motif discovery tools on a large collection of ChIP-seq and HT-SELEX datasets.

We present an improved, faster version of our Bayesian Markov model software, BaMMmotif2. However, they are more prone to overfit the data and to learn patterns merely correlated with rather than directly involved in TF binding. Models that can learn dependencies between positions, for instance, induced by DNA structure preferences, have yielded markedly improved predictions for most TFs on in vivo data. Motifs are commonly described by position weight matrices, which assume that each position contributes independently to the binding energy. Accurate models for predicting binding affinities are crucial for quantitatively understanding of transcriptional regulation.
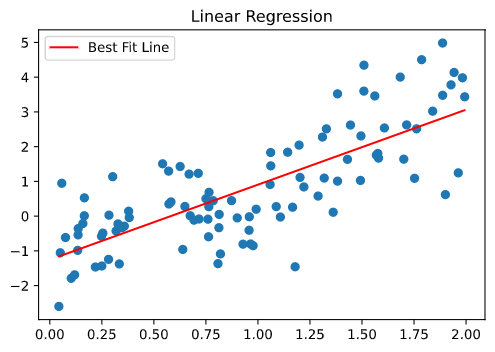
Obsolete.Transcription factors (TFs) regulate gene expression by binding to specific DNA motifs.
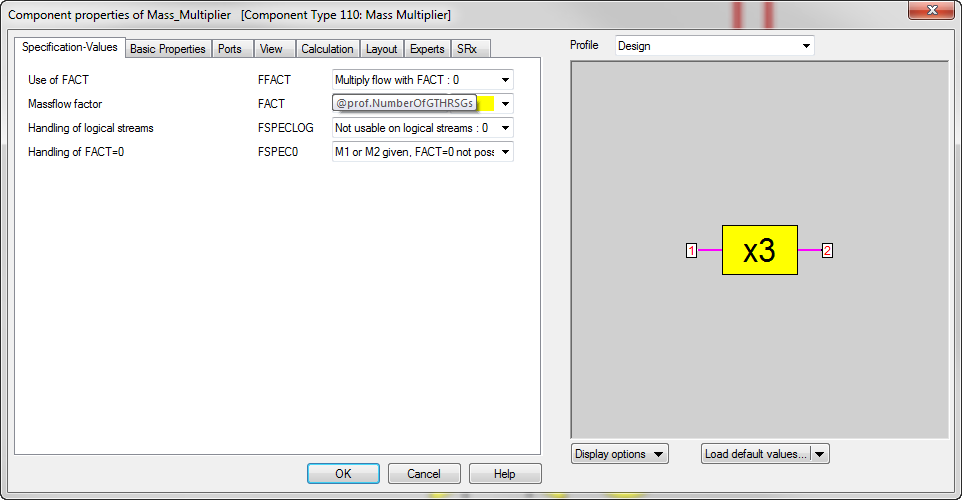
Statements Statements < Show List ) = EXPR Define a user definable function.
